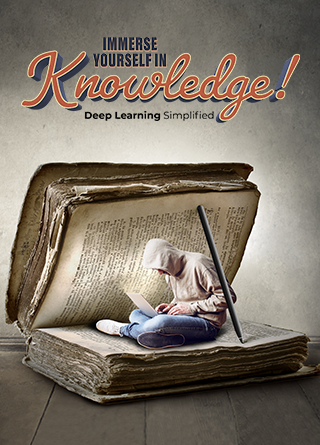
Emerging Technology
Deep Learning Simplified
By TechDogs Editorial Team
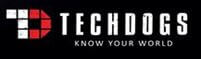
Overview
Coming to the real world, we bet you’ve asked yourself about the magical technology that enables driverless cars to distinguish a pedestrian from a streetlight - or the working of voice recognition in smartphones, TVs and virtual assistant devices such as Alexa?
The answer is Deep Learning. This tech has been getting loads of attention lately (and for good reason!). So, we decided to explain everything about Deep Learning in this blog.
If you’re interested in a comprehensive explainer with some Matrix puns sprinkled along, read on!
.png.aspx)
No, this is not a film review blog – but the Matrix movies have a lot of similarities with Deep Learning! We say that because they both rely on Artificial Intelligence and how it influences the lives of humans. The protagonist in the Matrix movies, Neo, is faced with the challenge of understanding and navigating a simulated reality created by sentient machines. What does this have to do with Deep Learning?
Well, Deep Learning can help computer systems mimic human intelligence. So, if you ever asked yourself how the machines created a virtual simulation, you now know the answer!
Deep Learning algorithms can learn and evolve over time, enabling machines to perform tasks that previously relied on human intelligence. It learns over time, hence the term “learning” in its name. This technology is a subset of Machine Learning and is essentially a Neural Network with several layers. (For a deep dive into Neural Networks, hop on to our explainer here!)
Deep Learning models learn how to perform classification tasks in images, text, or even video. They can achieve high levels of accuracy, sometimes exceeding human performance. (You can give up Neo, the machines are smarter!)
We bet your curiosity is piqued; let’s look at Deep Learning in more detail.
What Is Deep Learning?
Deep Learning is a specialized neural network. That means it is an algorithm that tries to recognize underlying relationships in data by imitating the human brain’s operation. The “deep” in Deep Learning refers to the depth of the neural network layers, or how many neural network layers it contains.
Traditional neural networks only contain 2-3 layers; Deep Learning networks can have tens of hundreds of layers! A single-layer neural network can make approximate predictions, however, stacking them together can help refine the accuracy. This is the basic idea behind the architecture of a Deep Learning network.
Don’t worry; we will look at terms such as “neural network” in detail. What is important is that Deep Learning drives everyday products and services, including digital voice assistants, credit card fraud detection, self-driving cars and many more. So, how did it get to be so awesome?
Let’s take a look!
Evolution And Origins Of Deep Learning
Deep Learning was not developed overnight; in fact, the seeds for the tech were sown over 60 years ago. Here’s a look at its timeline:
- Frank Rosenblatt developed and explored the basic concept of Deep Learning in his 1962 book, Principles of Neurodynamics. He introduced the idea of a multilayer perceptron with three layers: an input layer, a hidden layer and an output layer. This is the basic structure of modern Deep Learning models.
- The first working algorithm for a Deep Learning perceptron was published by Alexey Ivakhnenko and Lapa in 1967. This was a minor step, as the model did not use backpropagation (a term we will explain in the working of a Deep Learning model).
- Deep Learning architectures progressed with the development of convolutional neural networks (CNNs), introduced by Kunihiko Fukushima in 1980.
- The term “Deep Learning” was coined and introduced by Rina Dechter in 1986.
- In 1988, Wei Zhang et al. applied the backpropagation algorithm to a convolutional neural network for the first time. Their Deep Learning system was built for alphabet recognition.
- Then, in 1989, Yann LeCun et al. took it further and applied backpropagation to recognize handwritten ZIP codes on mail. While the algorithm worked, training took 3 days!
- In 2012, the ImageNet Large-Scale Visual Recognition Challenge was held where more than a million images had to be categorized using computer vision. Deep Learning was crowned as the undoubted winner. In the 2015 competition, it bettered the average human performance!
- In March 2019, Yoshua Bengio, Geoffrey Hinton and Yann LeCun were awarded the Turing Award for their breakthroughs in deep neural networks aka Deep Learning.
Today, Deep Learning is applied in diverse applications across domains. We mean, the same tech that allows self-driving cars to “see” can help AI assistants “hear” – how’s that possible?
How Does Deep Learning Work?
We know that diagram looks complex – but Deep Learning networks consist of multiple layers of interconnected nodes, each taking the inputs from the previous layer to refine and optimize the output. They consist of three layers:
-
Input Layer
This is where the Deep Learning model ingests the data for processing.
-
Output Layer
This is the final layer where the end prediction or classification is made and given as an output.
-
Hidden Layers
This is where the magic happens! As the computations progress from the input to the output, in a process called forward propagation, the weight of each node changes the values sent to the next layer slightly. This is carried out till the output reaches the final layer (output layer). Then, another process called backpropagation calculates the errors in predictions and adjusts the weights of the nodes as it moves backward from the output to the input layers to train the model. Together, forward propagation and backpropagation help a Deep Learning algorithm become accurate over time.
Imagine you want to build a Deep Learning system to recognize handwritten numbers. You input the numeral “1” (input layer) which progresses through the layers (forward propagation) till the model gives you a predicted value (output layer). Say the model identifies the number as “7” – don’t fret, the model can still learn. Deep Learning allows the model to change the weights of each node independently (backpropagation) till the output matches the input.
Viola! You have just trained in the Deep Learning algorithm.
Now, let’s see where it can be used!
Applications Of Deep Learning
Deep Learning can learn how to recognize elements in text, images and videos with high accuracy. Naturally, it has several applications:
-
Law Enforcement And Fraud Detection
Deep Learning algorithms can analyze transactional data and find patterns that suggest potential fraud or illegal activity. Additionally, it can parse emails, find words that might be used in phishing attempts and give you a likelihood of it being fraud.
-
Financial Services
Predictive analytics is powered by Deep Learning to allow financial institutions to perform algorithmic trading of stocks and assets, evaluate credit risk for loan approvals and optimize the management of client investment portfolios.
-
Customer Service
Deep Learning technologies are used by businesses in AI chatbots and other customer service applications. Traditional chatbots employ text recognition or Natural Language Processing capabilities. However, sophisticated AI chatbots can even recognize if a question has multiple possible answers and understand context and sentiment. Surely, you’ve heard of ChatGPT?
-
Image And Video Recognition
Deep Learning models can accurately and automatically classify objects, such as cars, people and faces, in images and videos. Uses include smart surveillance systems, self-driving cars and video search engines.
-
Virtual Assistants
The concept of chatbots is expanded by speech recognition in Siri, Amazon Alexa or Google Assistant, thanks to Deep Learning. This enables a unique and personalized way to engage with users.
-
Healthcare Diagnosis
The digitization of patient records has greatly benefited the healthcare sector, enabling medical professionals to use Deep Learning for analysis and evaluation before diagnosis. For example, it has been essential in analyzing medical scans to identify cancerous tumors.
What’s The Future Of Deep Learning?
Deep Learning became an overnight “star technology” when a robot beats a human player in the famed game of AlphaGo. Hey Neo, it can even defeat the evil machines from the Matrix!
Research into industry applications of Deep Learning will see higher interest and investment in the future, as it will become a “ubiquitous” presence in the industry. The focus will be on demonstrating faster learning from limited training materials, the ability to transfer learning and produce insights from half contexts, i.e., adaptive learning capabilities. This will allow businesses to deploy and reuse a Deep Learning system that has been trained on one task on a new one. With advanced hardware and processing software being developed, Deep Learning will get faster, more accurate and highly adaptable. We’re excited about the future, are you?
We Are At The Deep End Now!
We hope this isn’t you when someone talks about Deep Learning now!
This technology has immense potential to revolutionize the way we develop AI applications. It has already made tremendous progress in computer vision, natural language processing and image recognition. Deep Learning has the potential to simplify our lives and create a faster, smarter and more automated future! Excited?
Frequently Asked Questions
What is Deep Learning?
Deep Learning is a specialized form of neural network technology that imitates the human brain's operations to recognize underlying relationships in data. Unlike traditional neural networks, Deep Learning networks can have tens to hundreds of layers, allowing for more complex analysis and accurate predictions.
How Does Deep Learning Work?
Deep Learning networks consist of multiple layers of interconnected nodes, including input, hidden, and output layers. Through a process called forward propagation, data is ingested and processed through the layers to produce a prediction or classification. Backpropagation then adjusts the weights of nodes based on prediction errors, allowing the model to learn and improve over time.
What Are Some Applications of Deep Learning?
Deep Learning has diverse applications across various industries. It can be used for law enforcement and fraud detection by analyzing transactional data, for financial services in predictive analytics and algorithmic trading, for customer service through AI chatbots, and for image and video recognition in smart surveillance systems and self-driving cars. Additionally, it plays a crucial role in virtual assistants, healthcare diagnosis, and many more areas.
Enjoyed what you read? Great news – there’s a lot more to explore!
Dive into our content repository of the latest tech news, a diverse range of articles spanning introductory guides, product reviews, trends and more, along with engaging interviews, up-to-date AI blogs and hilarious tech memes!
Also explore our collection of branded insights via informative white papers, enlightening case studies, in-depth reports, educational videos and exciting events and webinars from leading global brands.
Head to the TechDogs homepage to Know Your World of technology today!
Disclaimer - Reference to any specific product, software or entity does not constitute an endorsement or recommendation by TechDogs nor should any data or content published be relied upon. The views expressed by TechDogs' members and guests are their own and their appearance on our site does not imply an endorsement of them or any entity they represent. Views and opinions expressed by TechDogs' Authors are those of the Authors and do not necessarily reflect the view of TechDogs or any of its officials. While we aim to provide valuable and helpful information, some content on TechDogs' site may not have been thoroughly reviewed for every detail or aspect. We encourage users to verify any information independently where necessary.
Trending Introductory Guides
Natural Language Processing (NLP) Software 101
By TechDogs Editorial Team
Everything You Need To Know About Quantum Networking
By TechDogs Editorial Team
A Comprehensive Guide To Smart Home Security
By TechDogs Editorial Team
Backup Your Business With Enterprise Backup Solutions
By TechDogs Editorial Team
A Simple Guide To Data Transformation
By TechDogs Editorial Team
Join Our Newsletter
Get weekly news, engaging articles, and career tips-all free!
By subscribing to our newsletter, you're cool with our terms and conditions and agree to our Privacy Policy.
Join The Discussion